2022 Research Review / DAY 1
A Machine Learning Pipeline for Deepfake Detection
The use of AI to create image or video forgeries (deepfakes) represents a pervasive threat to the DoD. Indeed, both the National Defense Authorization Act (NDAA) of 2021 and the Identifying Outputs of Generative Adversarial Networks (IOGAN) Act of 2020 address the risk posed by deepfakes to national security. However, the barriers to creating photorealistic deepfakes are low: They can be created with a single, affordable gaming-type graphics processing unit with easily accessible and frequently maintained open source software.
The aim of this project is to develop a deepfake detection prototype framework… with at least 85% accuracy…
Dr. Shannon GallagherMachine Learning Research Scientist
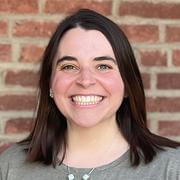
Fortunately, the neural network architectures used to create deepfakes can also be engineered to detect them. AI detection algorithms have been developed that can identify manipulation not readily visible to the human eye, but no detector tool exists that can ingest multiple modes of media (image, video) and process them at scales required in DoD or other medium-to-large scale environments. The aim of this project is to develop a deepfake detection prototype framework that incorporates open source and novel detector algorithms capable of detecting at least three types of AI artifacts per mode with at least 85% accuracy, for both image and video modes.
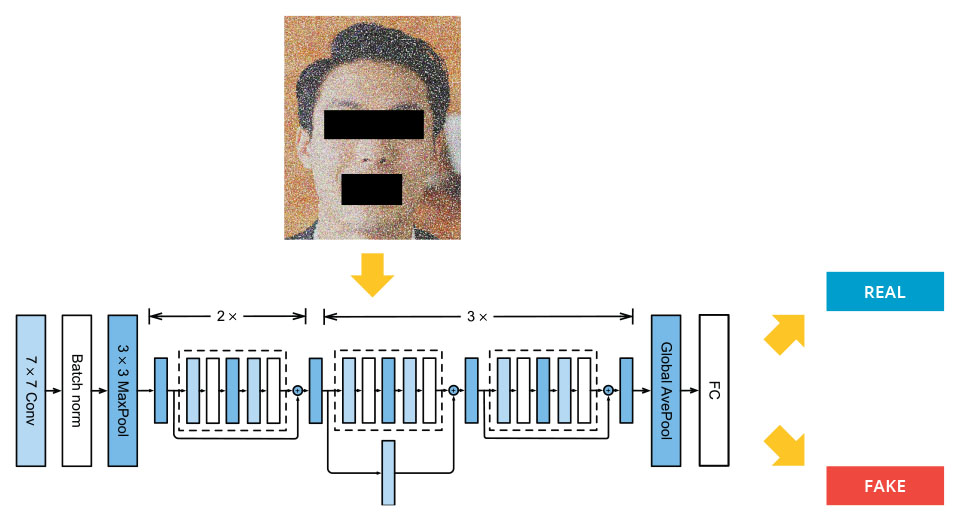
The initial phase of our work consisted of identifying and testing state-of-the-art, open source deepfake detector algorithms and selecting those that perform the best on open source data. We plan to incorporate these algorithms into a prototype tool that can ingest each mode of data (image and video) at scale, perform filtering and triaging relevant to a specific type of media (e.g., images of human faces, text, videos containing human subjects), and assign a probability of that media having been manipulated with AI. We will work to determine the best way to combine individual model scores into a robust, high-integrity performance metric. The goal of these efforts is a working prototype capable of ingesting all modes of data and able to detect at least three types of AI artifacts for each mode with at least 85% accuracy.
In Context
This FY2022–23 project
- aligns with the SEI technical objective to bring capabilities that make new missions possible or improve the likelihood of success of existing ones
- aligns with the DoD software strategy to realize computational and algorithmic advantage
- aligns with the DoD software strategy to accelerate the delivery and adoption of AI
Principal Investigator
Dr. Shannon Gallagher
Senior Architecture Researcher
SEI Collaborators
Dominic Ross
Multi-Media Design and Communications Lead
Jeffrey Mellon
Machine Learning Research Scientist
Dr. Catherine Bernaciak
Senior Machine Learning Research Scientist